
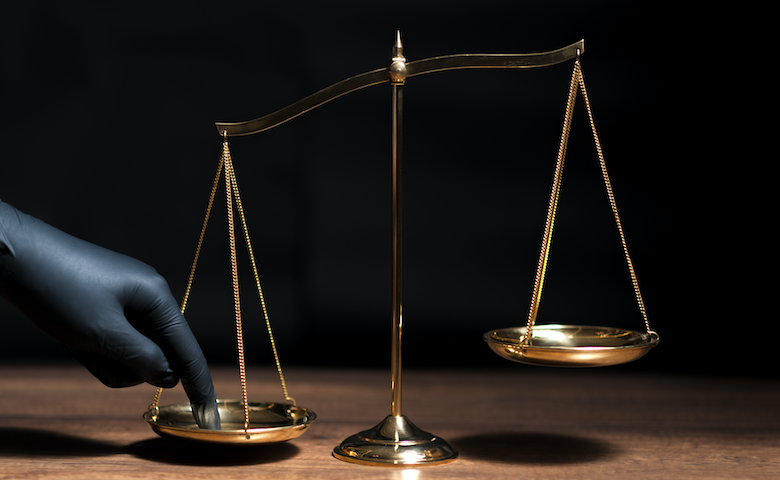
Selection bias is the term used to describe the situation where an analysis has been conducted among a subset of the data (a sample) with the goal of drawing conclusions about the population, but the resulting conclusions will likely be wrong (biased), because the subgroup differs from the population in some important way. Selection bias is usually introduced as an error with the sampling and having a selection for analysis that is not properly randomized.
Examples of selection bias
Perhaps the most well-known example of selection bias is the confirmation bias, whereby people tend to recall only examples that confirm their existing beliefs.
Another example is the phenomenon whereby people who are lucky when they first gamble assume incorrectly that this is a sign they will be lucky for the rest of their lives. It is believed that this makes such people more likely to become addicted to gambling.
Publication bias, whereby journals tend to publish only novel or interesting conclusions, means that published academic studies generally contain a selection bias, and this has been posited as a cause of the replicability crisis in science and research.
Types of selection bias
The most common type of selection bias in research or statistical analysis is a sample selection bias. A subgroup represents a sample of the population (e.g., a sample of people). In principle, the bias can occur through selection effects in other aspects of the research process, such as which variables to use in analysis, and which tools to use to perform measurement. In practice, nearly all examples of selection biases are variants of sample selection bias, relating to either how people are selected or how measurements are taken (i.e., time-based sampling). Read more to discover how to avoid sampling bias.
Worked example of selection bias
How selection bias works can be understood by looking at how it affects correlation. The chart below (which shows hypothetical data) seems to suggest that the correlation between beer consumption and whether or not people think that beer consumption causes brain damage is small (r = -0.1).
The analysis above is based only on people who consumed beer in the past seven days. It is unlikely that an analysis of the relationship between beer consumption and the perception that beer will cause brain damage based on people who consume beer regularly will be very reliable: presumably, people with concerns about brain damage and beer will consume less beer than those with no such concerns.
The chart below illustrates how you can have a strong correlation between two variables, but when a subgroup of the data is selected in such a way that the subgroup over- or under-represents aspects of the data, the conclusion can change dramatically.
How to avoid selection biases
Mechanisms for avoiding selection biases include:
- Using random methods when selecting subgroups from populations.
- Ensuring that the subgroups selected are equivalent to the population at large in terms of their key characteristics (this method is less of a protection than the first, since typically the key characteristics are not known).