
Unlock the Power of Text Analytics for Better Business Decisions
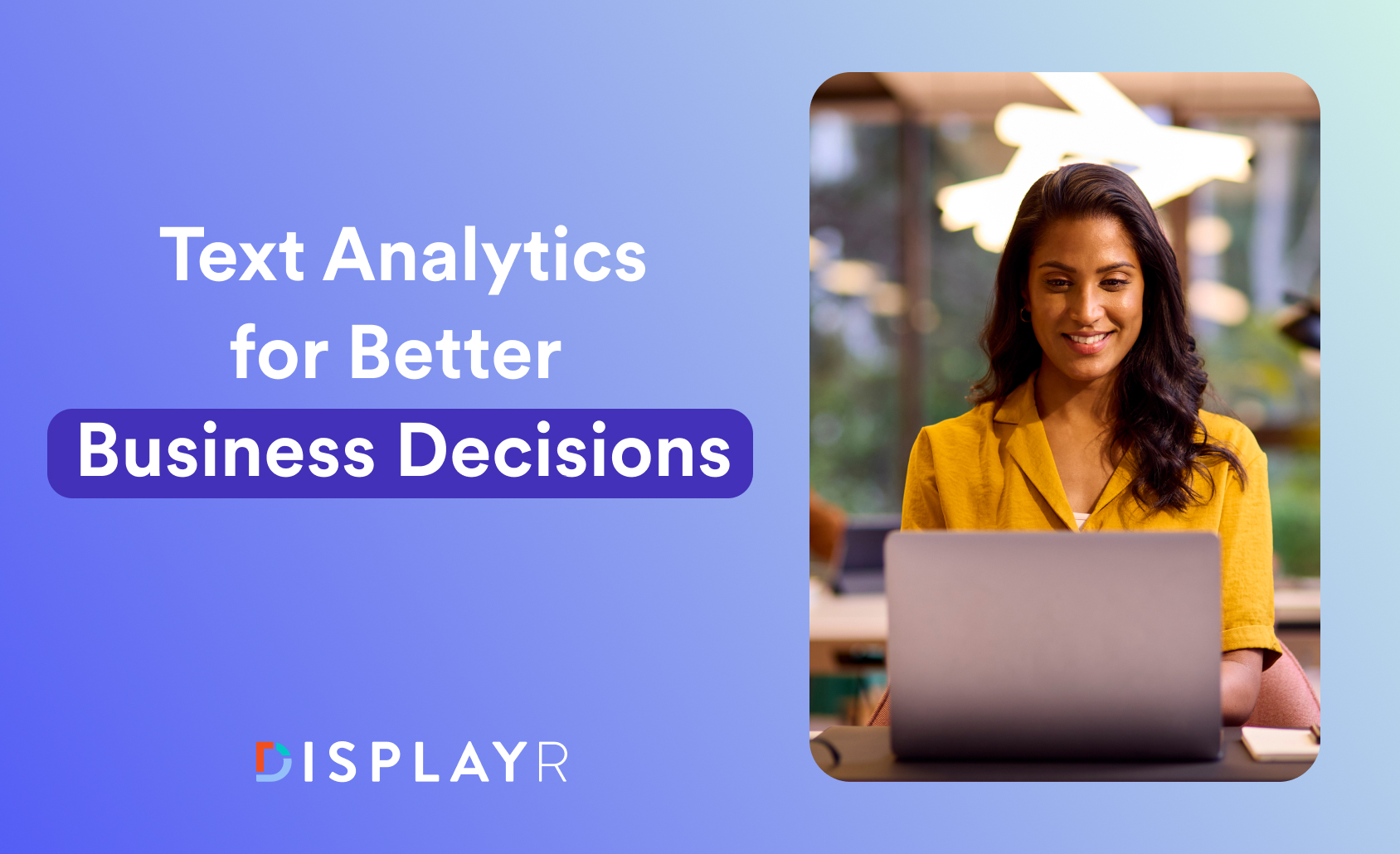
IntroductionIn today's climate, the most successful businesses use data to inform every decision they make. One valuable source of data is text—from employee feedback surveys to customer reviews, there are endless troves of textual data from which to glean insights.
Recent advances in AI text analytics have made it faster, cheaper, and easier than ever to turn unstructured textual data into business strategy. Here, we run through some examples of text analytics in the business world and show you how you can implement it yourself today.
Understanding Text Analytics
Text analytics has emerged as a crucial tool for businesses grappling with information overload in the digital age. While many use the terms text analytics and text mining interchangeably, they serve distinct purposes in the data analysis landscape. Text mining focuses on deriving qualitative insights from unstructured text, whereas text analytics provides quantitative results that can directly inform business decisions.
Natural Language Processing (NLP) forms the backbone of modern text analytics systems. These sophisticated tools employ semantic software and knowledge models to decode the nuances of human language. By transforming unstructured text into structured data, text analytics solutions help organizations overcome the inherent ambiguity in language.
Consider the word "apple" - it could refer to the fruit, the technology company, or even a person's name. This simple example demonstrates why context is crucial in text analysis. Modern text analytics platforms use several techniques to establish context:
- Classification of document types and content
- Entity identification within text
- Fact extraction from complex documents
- Relationship mapping between different textual elements
The power of text analytics lies in its ability to process vast amounts of textual data at scale. Organizations can analyze millions of documents, social media posts, customer reviews, and internal communications in a fraction of the time it would take human analysts.
Applications of Text Analytics Across Industries
Business intelligence represents one of the most valuable applications of text analytics. Companies leverage these tools to extract actionable insights from customer feedback, market research, and competitor analysis. Through sophisticated pattern recognition, text analytics can reveal trends that might otherwise remain hidden in massive datasets.
Healthcare organizations have found particular value in text analytics for processing patient records and medical research, while financial sector businesses use text analytics for risk assessments, fraud detection, regulatory compliance and market sentiment analysis.
Government agencies utilize text analytics to process citizen feedback and monitor social media for public safety concerns. For example, during natural disasters, text analytics helps emergency response teams identify areas requiring immediate assistance by analyzing social media posts and emergency calls.
E-commerce platforms have revolutionized their search capabilities through text analytics. Advanced algorithms now understand user intent beyond simple keyword matching, delivering more relevant results and improving customer satisfaction.
Techniques and Use Cases in Text Analytics
Sentiment analysis stands as one of the most widely adopted text analytics techniques. This approach examines text to determine the emotional tone and attitude expressed within it. Major brands use sentiment analysis to track public perception across social media, news articles, and customer reviews.
Topic modeling represents another powerful technique in the text analytics arsenal. This method automatically identifies main themes within large document collections, helping organizations understand trends and patterns in their data. A leading research institution used topic modeling to analyze 50 years of scientific publications, revealing previously unknown connections between different fields of study.
Named Entity Recognition (NER) serves as a fundamental building block for many text analytics applications. This technique identifies and classifies named entities such as:
- People and organizations
- Locations and facilities
- Dates and time expressions
- Monetary values and quantities
Event extraction takes text analytics further by identifying specific events mentioned in content. Financial institutions use this technique to monitor news feeds for market-moving events, while intelligence agencies employ it to track global developments.
Sentiment Analysis in Practice
Sentiment analysis has evolved beyond simple positive/negative classification. Modern systems (like Displayr) can detect subtle emotional nuances, sarcasm, and context-dependent meanings. Companies now use multi-dimensional sentiment analysis to understand:
- Customer satisfaction levels
- Brand loyalty indicators
- Purchase intent signals
- Service quality perception
The technology sector has embraced sentiment analysis for product development. Software companies monitor developer forums and social media to:
- Track feature requests
- Identify bug reports
- Gauge adoption rates
- Measure developer satisfaction
These insights help prioritize development efforts and improve product roadmap planning.
Ready to use text analytics to inform your business strategy?
Start a free trial of Displayr.
Enhancing Text Analytics with AI
AI is a top priority for many business leaders seeking its transformative potential. However, generative AI like ChatGPT presents challenges around data cutoffs, potential biases, and ethical issues. This is where text analytics can complement AI by adding more precision and transparency.
- Text analytics solutions work with specific enterprise vocabularies and taxonomies. This brings structure and governance compared to freestyle generative AI.
- The future is integration of text analytics and generative AI like GPT and other large language models. Text analytics can provide the base data set for AI to generate insights.
Practical Use Cases
Some practical use cases for text analytics for businesses include:
Identifying Key Themes
- Analyze customer feedback to identify and highlight predominant themes. This provides insight into top-of-mind topics and concerns.
- Gather social media posts and analyze them to identify trending topics related to the industry. This reveals rising issues and opportunities.
Understanding Pain Points
- Extract the most common pain points mentioned in customer support tickets. Identify opportunities to improve products, services, and documentation.
- Scan industry forums and discussions to find unmet needs or desires. Use this input for product development.
Crisis Monitoring
- Track and analyze sentiment of online mentions during a PR crisis. Gauge how public opinion is evolving and when it's safe to resume normal marketing.
Competitive Intelligence
- Monitor competitor press releases and announcements. Identify key messaging and positioning in their go-to-market strategy.
Text Analytics Process
Conducting text analytics involves several key steps:
Data Gathering
First, relevant text data needs to be collected from internal databases, surveys, call center logs, emails, chats, social media, and other sources. Both structured and unstructured data can be valuable.
Data Preparation
The raw text data then goes through preparation steps:
- Tokenization: breaking text into individual words, phrases, or symbols.
- Part-of-speech tagging: labeling words with their type and function.
- Parsing: analyzing sentence structure and relationships.
- Lemmatization: grouping words into root forms.
- Stemming: reducing words to their base form.
Stopwords like "a", "and", "the" are also removed to focus on keywords.
Text Analytics Techniques
Prepared data can then be analyzed using techniques like:
- Text classification: categorizing text into pre-defined topics.
- Text extraction: identifying and pulling out key pieces of information.
- Sentiment analysis: detecting emotional tone as positive, negative or neutral.
Data Visualization
Finally, the outputs of text analytics are visualized using charts, plots, tables, infographics or dashboards. This enables businesses to spot trends, patterns, and insights at a glance.
Make Text Analytics Work for Your Business
No matter what industry you work in, text analytics can help you understand your data to inform better decision-making. With Displayr, you can take the hard work out of text analysis. Rather than manually coding and reading through thousands of verbatims, you can direct all of your focus toward finding answers in your text data.